
- This event has passed.
GeoAI Speaker Series – May 15, 2024
May 15, 2024 @ 2:00 pm - 3:00 pm
Discover groundbreaking research and applications at the intersection of Artificial Intelligence (AI) and geospatial technology with the OGC GeoAI Speaker Series. Each online session features a 30-minute presentation from an AI expert, followed by a moderated question and answer period. These conversations will help the AI community overcome challenges and identify new opportunities to build the future of location technology for the good of society. The GeoAI speaker series is open to both OGC Members and the general public.
Title
Knowledge-Guided Machine Learning: A New Framework for Accelerating Scientific Discovery and Addressing Global Environmental Challenges
Abstract
Climate change, loss of bio-diversity, food/water/energy security for the growing population of the world are some of the greatest environmental challenges that are facing the humanity. These challenges have been traditionally studied by science and engineering communities via process-guided models that are grounded in scientific theories. Motivated by phenomenal success of Machine Learning (ML) in advancing areas such as computer vision and language modeling, there is a growing excitement in the scientific communities to harness the power of machine learning to address these societal chal-lenges. In particular, massive amount of data about Earth and its environment is now continuously be-ing generated by a large number of Earth observing satellites, in-situ sensors as well as physics-based models. These information-rich datasets in conjunction with recent ML advances offer huge potential for understanding how the Earth’s climate and ecosystem have been changing, how they are being impacted by humans actions, and for devising policies to manage them in a sustainable fashion. However, capturing this potential is contingent on a paradigm shift in data-intensive scientific discovery since the “black box” ML models often fail to generalize to scenarios not seen in the data used for training and produce results that are not consistent with scientific understanding of the phenomena.
This talk presents an overview of a new generation of machine learning algorithms, where scientific knowledge is deeply integrated in the design and training of machine learning models to accelerate scientific discovery. These knowledge-guided machine learning (KGML) techniques are fundamentally more powerful than standard machine learning approaches, and are particularly relevant for scientific and engineering problems that are traditionally addressed via process-guided (also called mechanistic or first principle-based) models, but whose solutions are hampered by incomplete or inaccurate knowledge of physics or underlying processes. While this talk will illustrate the potential of the KGML paradigm in the context of environmental problems (e.g., Ecology, Hydrology, Agronomy, climate science), the paradigm has the potential to greatly advance the pace of discovery in any discipline where mechanistic models are used.
Speaker
Dr. Vipin Kumar
Vipin Kumar is a Regents Professor and holds William Norris Chair in the department of Computer Science and Engineering at the University of Minnesota. His research spans data mining, high-performance computing, and their applications in Climate/Ecosystems and health care. He also served as the Director of Army High Performance Computing Research Center (AHPCRC) from 1998 to 2005. He has authored over 400 research articles, and co-edited or coauthored 11 books including two widely used text books “Introduction to Parallel Computing”, “Introduction to Data Mining”, and a recent edited collection, “Knowledge Guided Machine Learning”. Kumar’s current major research focus is on knowledge-guided machine learning and its applications to understanding the impact of human induced changes on the Earth and its environment.
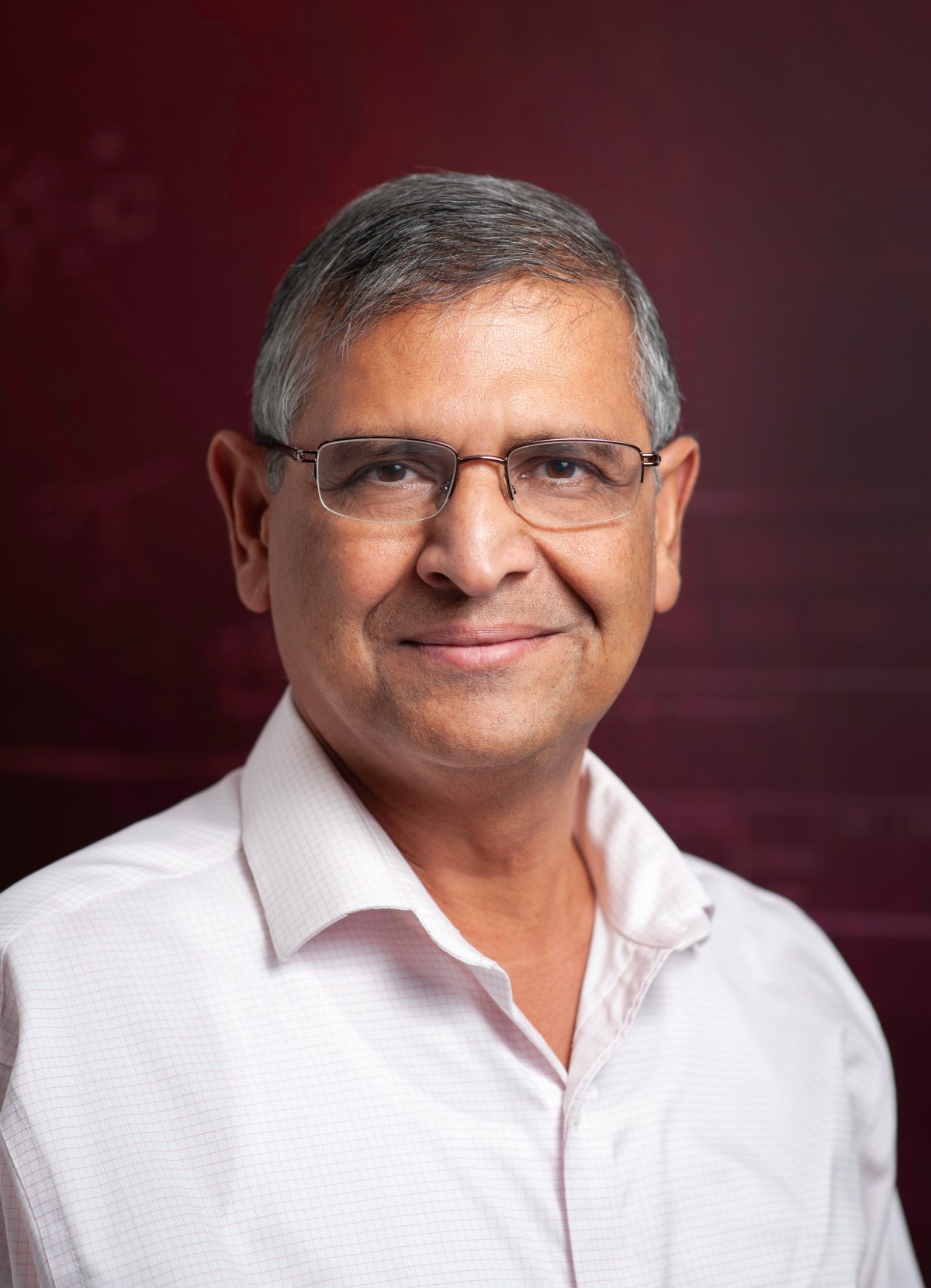