Far from being a “sci-fi” tech, Artificial Intelligence (AI) already plays a crucial role in many domains and is revolutionizing existing technologies. During the last decade, AI techniques such as Machine Learning (ML) and especially Deep Learning (DL), have improved significantly due to an abundance of data coupled with advancements in high-performance computing. As with so many technology domains, these new AI capabilities have reoriented and transformed GIS and Remote Sensing, providing new solutions and greatly increasing efficiency. The application of AI technologies to solve problems experienced by the geospatial community has become known as “GeoAI.”
The geospatial science community, for example, commonly uses AI and related techniques to better harness the otherwise insurmountable volume of Earth Observation (EO) data being created for geospatial analysis across various domains – such as smart cities, environmental management, and disaster management. However, the possible applications for GeoAI are only just beginning to surface.
“I would define GeoAI as a set of methods or automated entities that use geospatial data for perceiving, constructing (automating), and optimizing spaces in which humans – as well as everything else – can safely and efficiently continue their geographically referenced activities,” said Kyoung-Sook Kim, a co-chair of the OGC GeoAI Domain Working Group.
“GeoAI can bring significant benefits to drive the next generation of service innovation in many applications, including autonomous transportation, sustainable smart city planning/implementation, augmented building and energy management, self-optimized manufacturing, epidemic outbreak prediction, personal experience augmentation, and more. However, it also faces a variety of new ethical, legal, social, and technological challenges. I believe that international standards will play a pivotal role in ensuring widespread interoperability and security benefits among the various disciplines dealing with AI.”
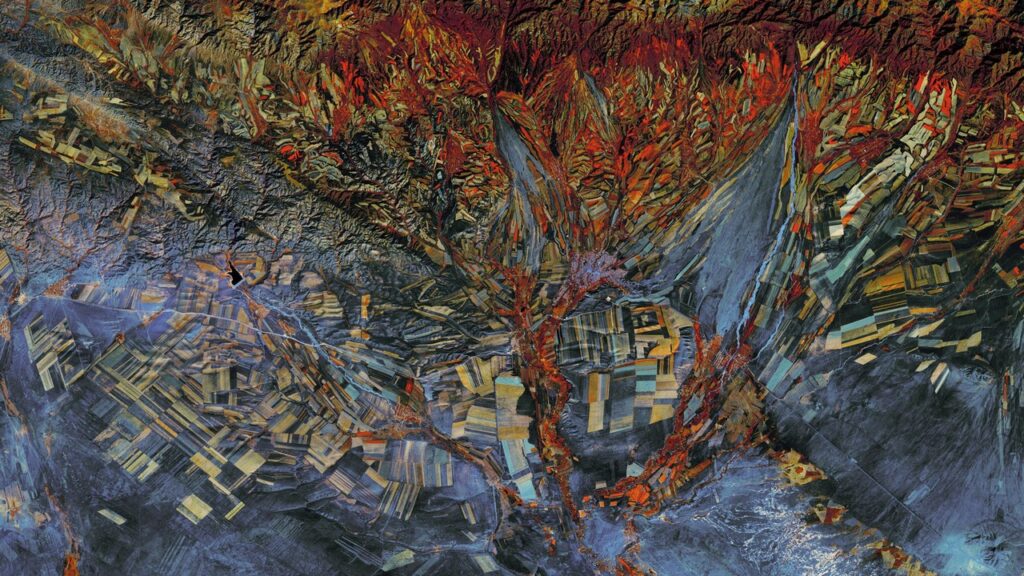
Innovations in GeoAI powered by open standards have lower development and implementation costs, reach the market sooner, and enable seamless horizontal and vertical integration/composition of GeoAI systems. Standards will also increase the safety of the computational approaches and algorithmic techniques that power the insights provided by AI engines.
With these benefits in mind, in 2018, OGC formed the Artificial Intelligence in Geoinformatics Domain Working Group (better known as the GeoAI DWG) to identify use-cases and applications related to AI in geospatial applications. The GeoAI DWG provides an open forum for broad discussion and presentation of use-cases for AI and its related technologies in the geospatial domain, with the purpose of bringing geoscientists, computer scientists, engineers, entrepreneurs, and decision makers from academia, industry, and government together to develop, share, and research the latest trends, successes, challenges, and opportunities in the field.
The GeoAI DWG is additionally tasked with investigating the feasibility and interoperability of OGC standards to support the use and re-use of geospatial data in AI applications, as well as describe gaps and issues that could lead to new geospatial standards.
“When we proposed the OGC GeoAI DWG, we found three main issues in applying AI technologies to geospatial domains. First, there are few large-scale benchmark training datasets like ImageNet, which is used for object recognition in computer vision projects. Even though there is a massive amount of satellite imagery and point cloud data available, most of them are not ready to use for geospatial Machine Learning tasks,” said Kyoung-Sook.
“Second, compared to the related fields of image processing and natural language processing, few open tools and workflows using GeoAI are shared in GIS and other business operations,” said Kyoung-Sook. “Organizations still pay for the full development and implementation cost when adapting a new GeoAI technique into a business case, rather than building upon established open tools and solutions.
“The third issue, which I think is the most important, is supporting trustworthy and safe GeoAI technology to support both the Earth’s and humanity’s well-being. The 2018 report from the World Economic Forum (Fourth Industrial Revolution for the Earth Series – Harnessing Artificial Intelligence for the Earth) states that the most important consideration in the development of AI, regardless of the AI stage, is to ensure sustainable benefits for humanity, which include being both ‘human-friendly’ and ‘Earth-friendly.’
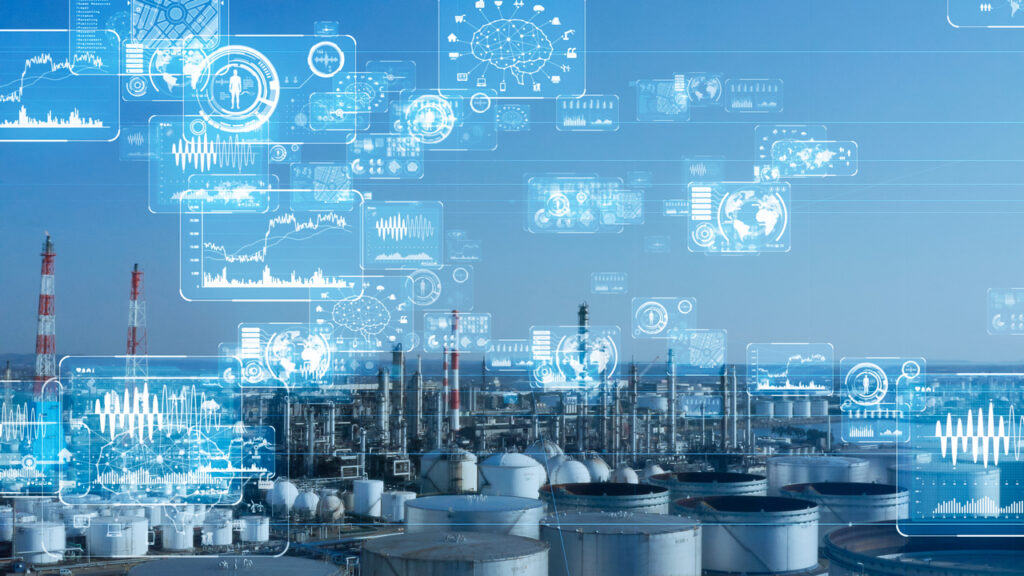
“Going back to the first issue, I would like the GeoAI DWG to first solve the lack of training datasets and improve the sharing of knowledge from skilled people. This lack of data and knowledge-sharing causes misuse of AI and creates biased AI models. To address this, the DWG members have started to collect and analyze AI-related applications and use-cases in our communities.
“Recently, OGC has also started the formation of the new Sample Markup Language for Artificial Intelligence Machine Learning Standards Working Group (SampleML-AI/ML SWG) to develop a standard for documenting, storing, and sharing the geospatial sample data.”
A key component of ML techniques and processes is the use of sample data – data with known provenance, consistent metadata, and quality measurements – to consistently tune and train ML applications. The lack of consistent and known sample data is hindering advanced EO science applications, causing reproducibility issues, and making it difficult to compare results across studies.
Sample data should have sufficient metadata in a machine-readable standard format, and include general spatiotemporal information and sample data-specific attributes to facilitate data discovery and query. Due to their utility in Geospatial ML applications, many academic and industrial areas have focused on creating their own benchmark datasets.
However, in order to access and share these training datasets easily and effectively, an international standard for data schema and formats is required. One solution proposed by OGC is to develop a standardized Sample Markup Language for AI/ML – based on commonly used industry standards wherever possible – that allows users to document, store, and share geospatial sample data over the web following the FAIR data management principles of Findability, Accessibility, Interoperability, and Reusability.
The formation of the SampleML-AI/ML Standards Working Group, endorsed by the GeoAI DWG, is nearly complete. The working group will then be tasked with developing an OGC SampleML-AI/ML Standard, with initial geospatial sample data categories for remote sensing imagery, moving features (typically vehicle trajectories), and related spatial content.
Further to this, OGC’s Testbed 18 has a task dedicated to Machine Learning Training Datasets. To quote the Testbed 18 Call For Participation: “The goal of this Testbed-18 task is to develop the foundation for future standardization of TDS for Earth Observation applications. The task shall evaluate the status quo of training data formats, metadata models, and general questions of sharing and re-use. Several initiatives, such as ESA’s AI-Ready EO Training Datasets (AIREO) have developed suggestions that could be used for future standardization. Other initiatives focused on the development of training data repositories, such as the Radiant MLHub, an open-access geospatial training data repository where anyone can discover and download ML-ready training datasets.” To learn more about this task, see section 2.2. Machine Learning Training Datasets in the Testbed-18 Call For Participation.
GeoAI is already doing incredible things for the location industry. However, to truly reach its potential, we need to build a strong foundation of open standards for sharing sample data and creating open tools and workflows, and open best practices for ensuring the safe and ethical use of this powerful technology.
If you’re interested in contributing to creating standards, best-practice, and/or documenting use-cases for GeoAI applications, consider joining the Artificial Intelligence in Geoinformatics Domain Working Group. OGC Members can join via the OGC Portal here. Non-members are welcomed to join by contacting OGC using the form at ogc.org/contact.
Interested parties are also encouraged to attend the next OGC Member Meeting, where the OGC GeoAI DWG will likely meet (TBC), along with many other related OGC Standards and Domain Working Groups.
A version of this article originally appeared in the Autumn 2021 issue of GeoConnexion International Magazine.